Written by Audrey Way, Data Visualization Specialist, Illumination Works
Despite all the data at our fingertips, businesses still struggle to turn insights into action. The problem isn’t the numbers—it’s how our brains interpret them, and without integrating decision science and psychology, even the best analytics can lead to poor decisions.
Designing BI/BA for the Way Humans Think
The Key to Effective Analytics. Business intelligence (BI) and business analytics (BA) promise to transform raw data into actionable insights. Yet, despite cutting-edge dashboards and AI-powered analytics, many organizations still struggle to translate data into better decisions. Why? Because data alone isn’t enough. In fact, 41% of business leaders cite a lack of understanding due to data complexity or inaccessibility (1).
Numbers, charts, and key performance indicators don’t make decisions—people do. And people don’t think like machines. We are influenced by cognitive biases, emotions, and mental aspects that shape how we interpret and act on data. To build truly effective BI/BA solutions, we must integrate principles from decision science and human psychology—disciplines that reveal how people actually process information and make choices.
In this article we will discuss harnessing the power of data and the hidden forces driving our choices as well as review decision-making models supported by BI/BA and the future of decision intelligence.
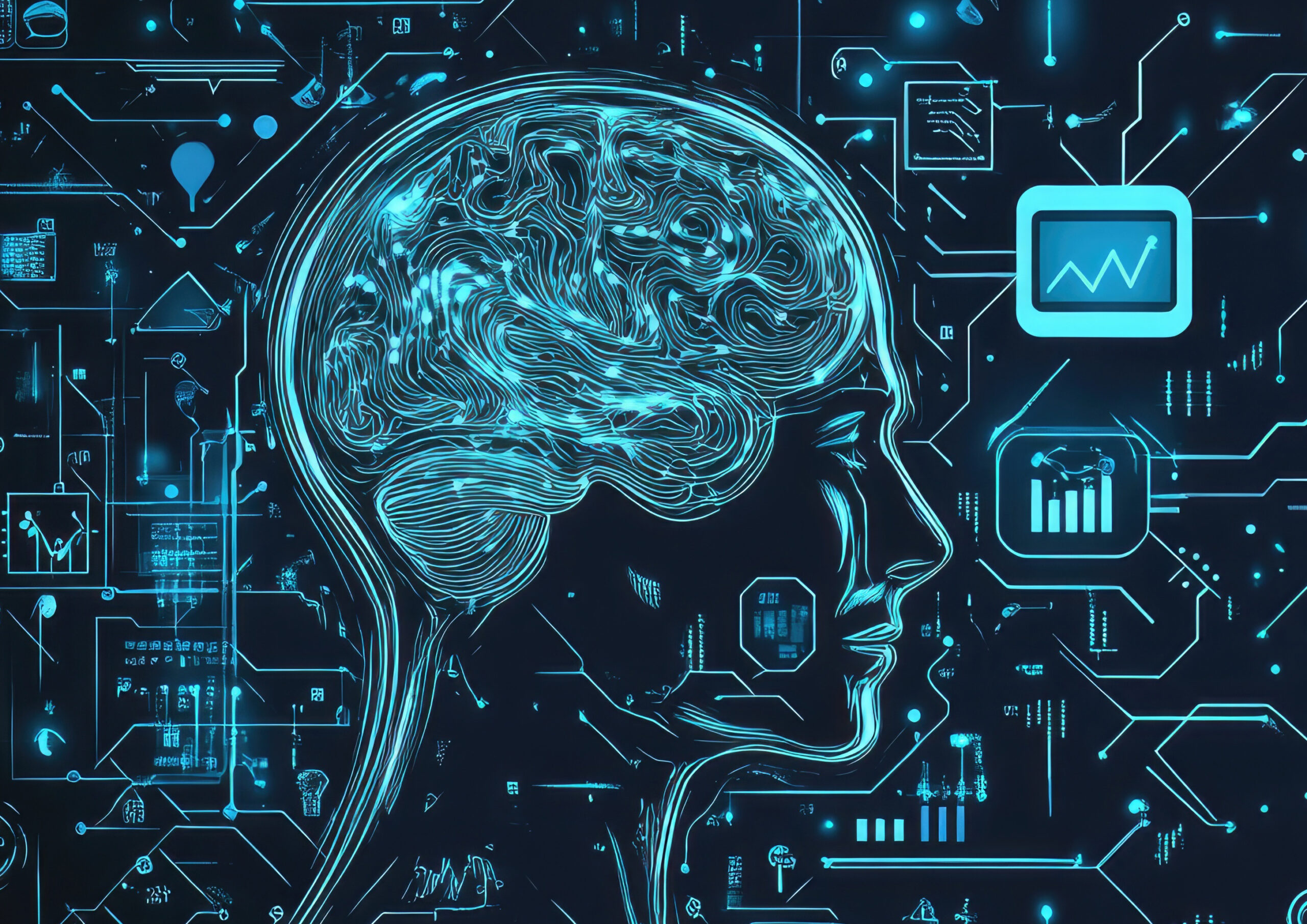
Harnessing the Power of Data
Intelligent Analytics. IBM defines business intelligence as descriptive, focusing on analyzing past and present data to answer What happened? and What is happening now? Whereas, business analytics is predictive and prescriptive, using data modeling to answer What will happen? and What should we do about it? (2) The two overlap in the present, where historical data is analyzed to predict future trends.BI/BA is not just about delivering data but structuring it in a way that aligns with human thought processes. Data science and psychology need to be married together in order to make the most significant impact.
BI and BA are increasingly converging as businesses demand more intelligent, real-time, and forward-looking insights. While BI focuses on monitoring, reporting, and diagnosing, BA expands into predicting and optimizing outcomes. Together, they create a powerful ecosystem for data-driven business strategies.
However, answering these critical questions also requires robust data science and modeling techniques operating behind the scenes. These underlying methodologies form the foundation for BI/BA tools, which then translate complex computations into accessible visualizations for reports and dashboards.
Traditional BI/BA tools focus on delivering data, but without considering how users interpret and act on it, insights can be misused or ignored. By leveraging cognitive science, we can design analytics that align with human decision-making patterns, reduce bias, and drive smarter business outcomes. Effective BI/BA isn’t just about reports and dashboards; it must also account for how humans process information and make decisions.
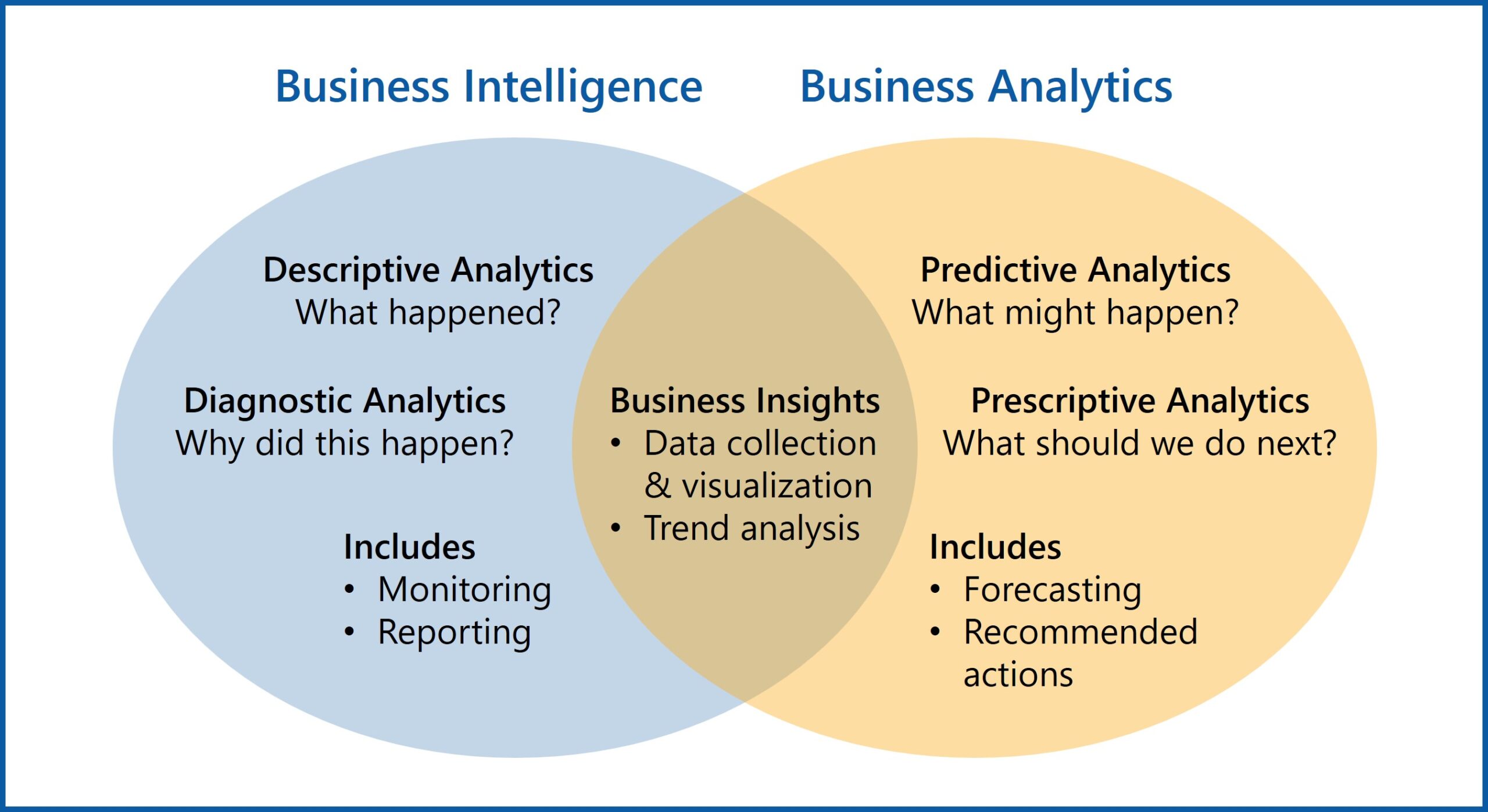
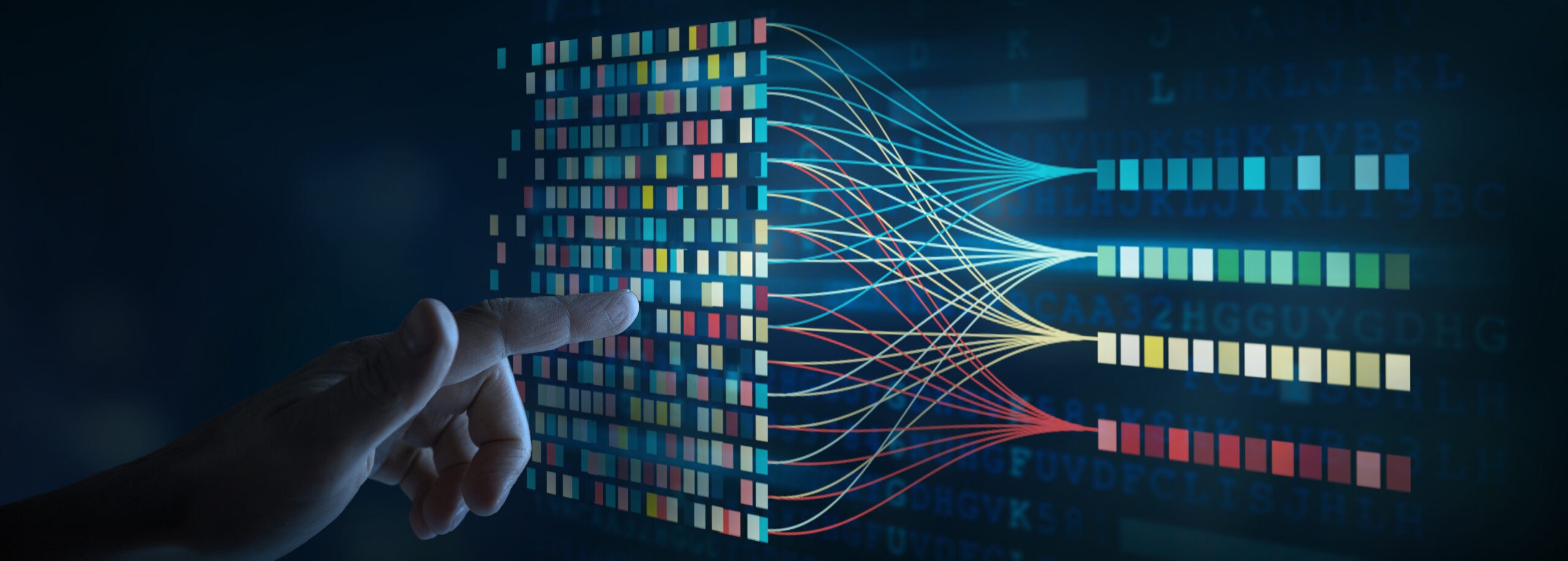
Analytics is more than dashboards and AI-powered analytics; it’s about bridging data and human decision making to unlock real business value.
“Although business intelligence does not tell business users what to do or what will happen if they take a certain course, neither is BI solely about generating reports. Rather, BI offers a way for people to examine data to understand trends and derive insights by streamlining the effort needed to search for, merge, and query the data necessary to make sound business decisions.” (3)
To build truly effective BI/BA solutions, we must integrate principles from decision science and human psychology.
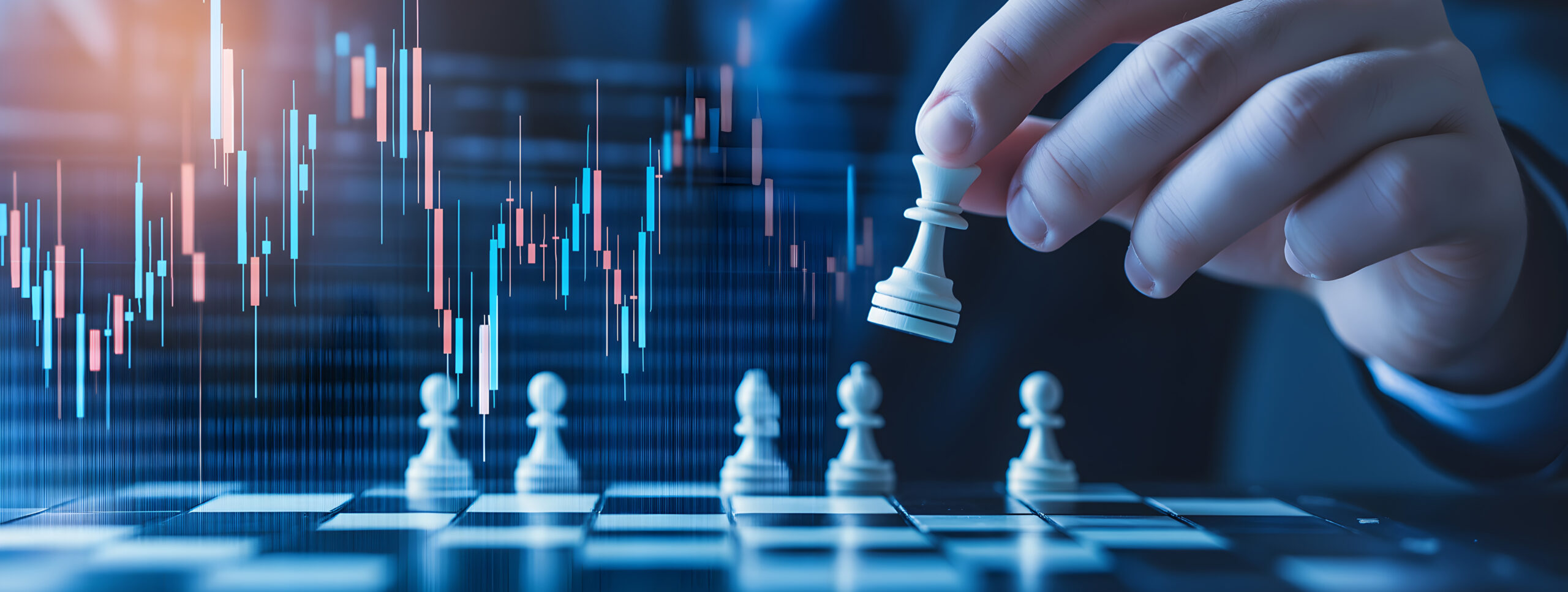
The Hidden Forces Driving Our Choices
Where Data Meets Human Judgement. Decision making isn’t simple any more. There is consensus that decision making is more complex than it has ever been, which makes it all the more important to consider how humans make decisions when presenting BI/BA solutions (4).
In the article Bringing Advanced Technology to Strategic Decision-Making: The Decision Intelligence/Data Science (DI/DS) Integration framework, Pratt et al. consider the many factors that come into play when making a decision, some conscious and some unconscious (4). There are internal and external influencers, assumptions, choices/actions, intermediaries, goals/outcomes, and dependencies and all of these can change to a certain degree. Furthermore, there is the decision-making thought process versus the implementation process, which may lead to disconnects between the predicted decision or outcomes and the actual decision, forcing the decision maker to pivot mid process.
If human intuition and judgement are woven into data-driven solutions, then technology can more effectively guide decision making.
Choices are the thoughts that become actions once the choices are executed. Intermediaries are the consequences of an action, while dependencies are how one factor influences another. All these decision parts lead to goals or outcomes.
Goals are conditions of an outcome, while outcomes are the accumulation of actions associated with a decision. Outcomes can be measured, while goals produce a true/false result. (5)
Ultimately, analyzing the anatomy of a decision helps BI/BA developers break down the factors that shape business choices, leading to more insightful analysis and impactful reporting. By distinguishing between decision influencers, a BI/BA can better contextualize decision making environments, identifying which factors can be controlled and which must be accounted for as assumptions. Understanding how choices, intermediaries, and dependencies interact ensures that reports go beyond surface-level trends and observations, revealing deeper patterns and relationships that drive business outcomes.
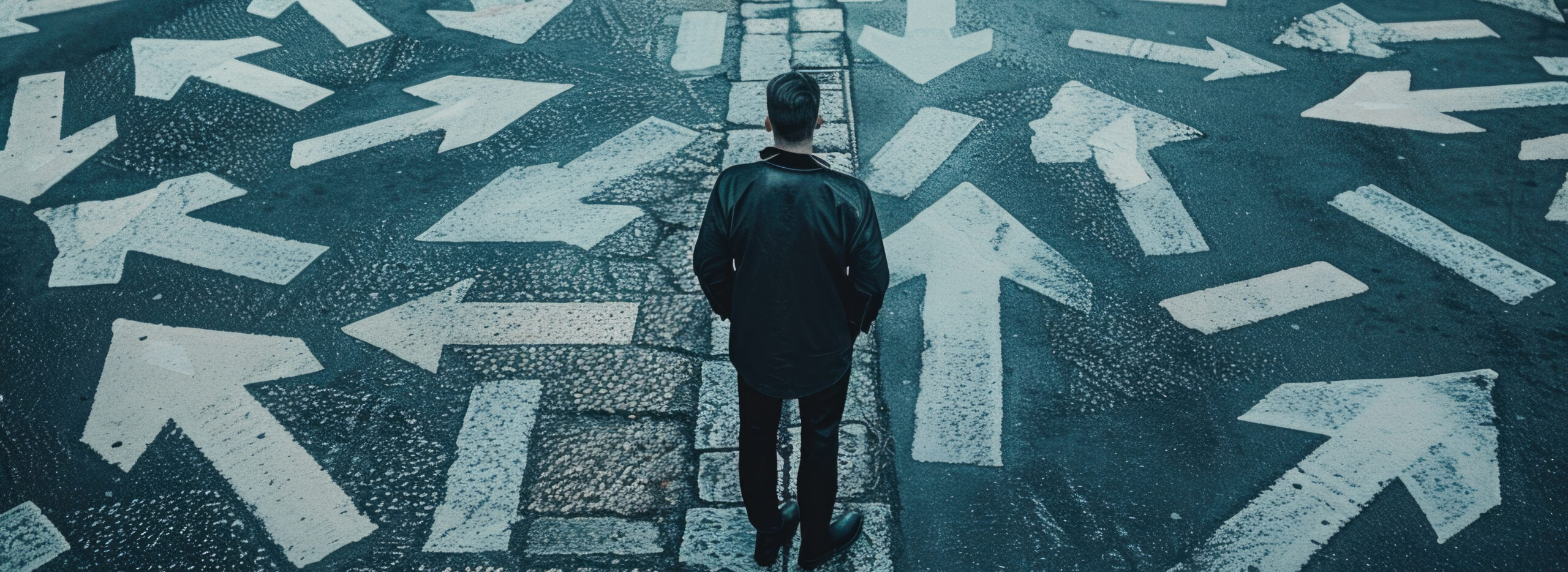
Decision-Making Models Supported by BI/BA
Bridging Data and Decision Science. Decision making can be approached in different ways, with three primary models supported by BI/BA—pattern recognition, rational, and bounded rationality—each offering a unique perspective on how choices are made in various contexts. In the article titled The Psychology of Decision-Making: How We Make Choices, Psychreg helps us define and understand these models. (5)
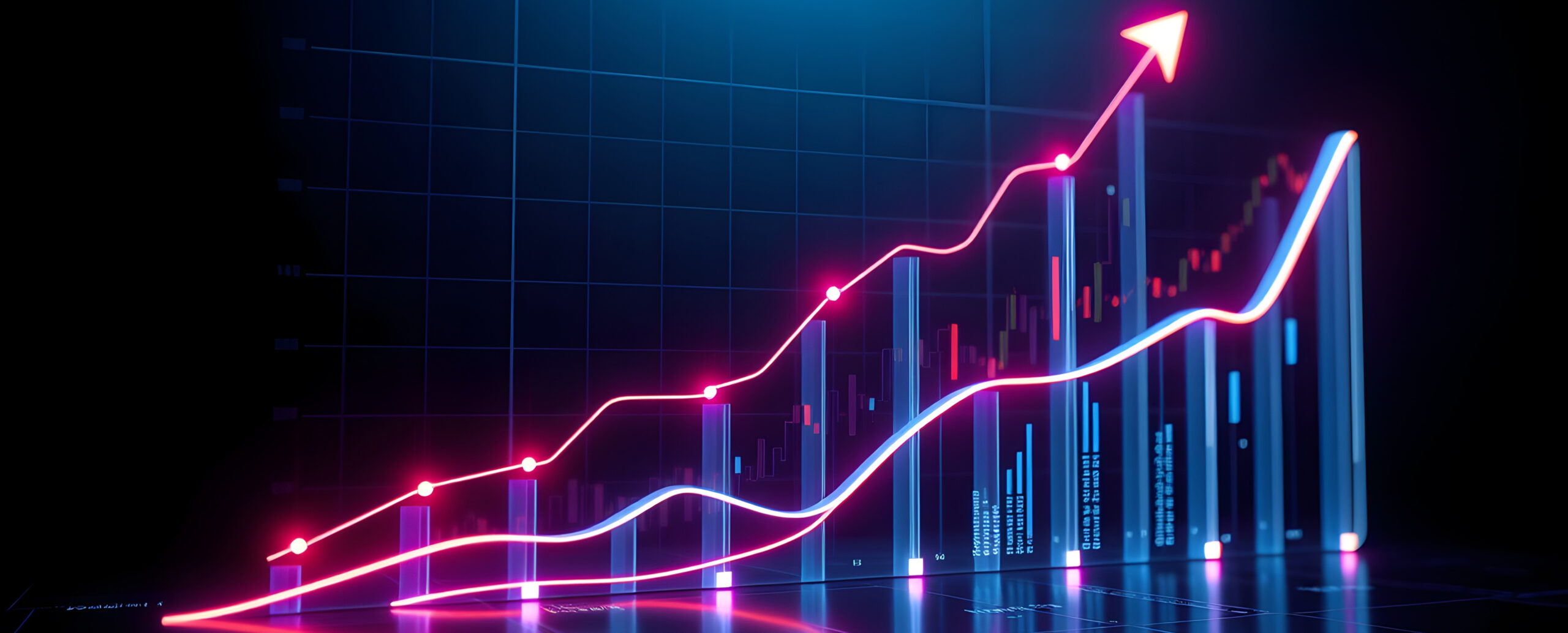
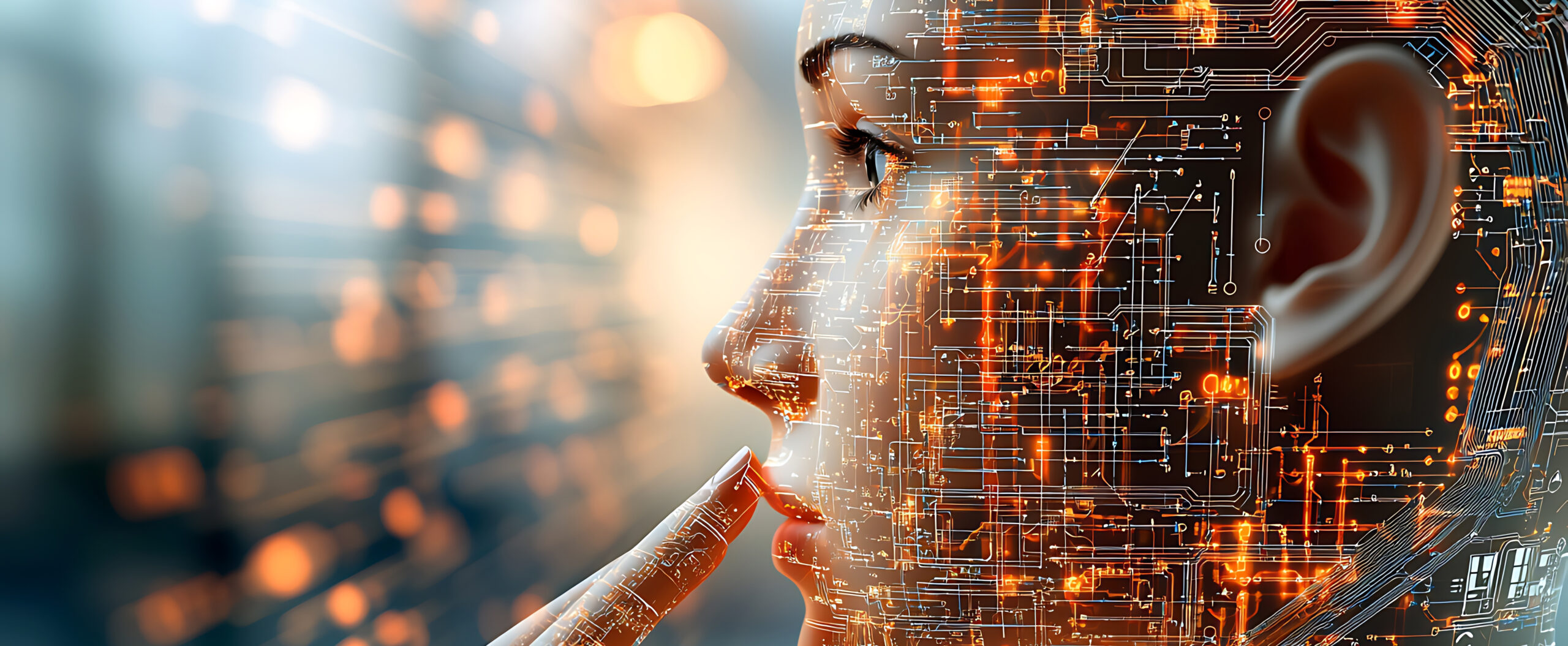
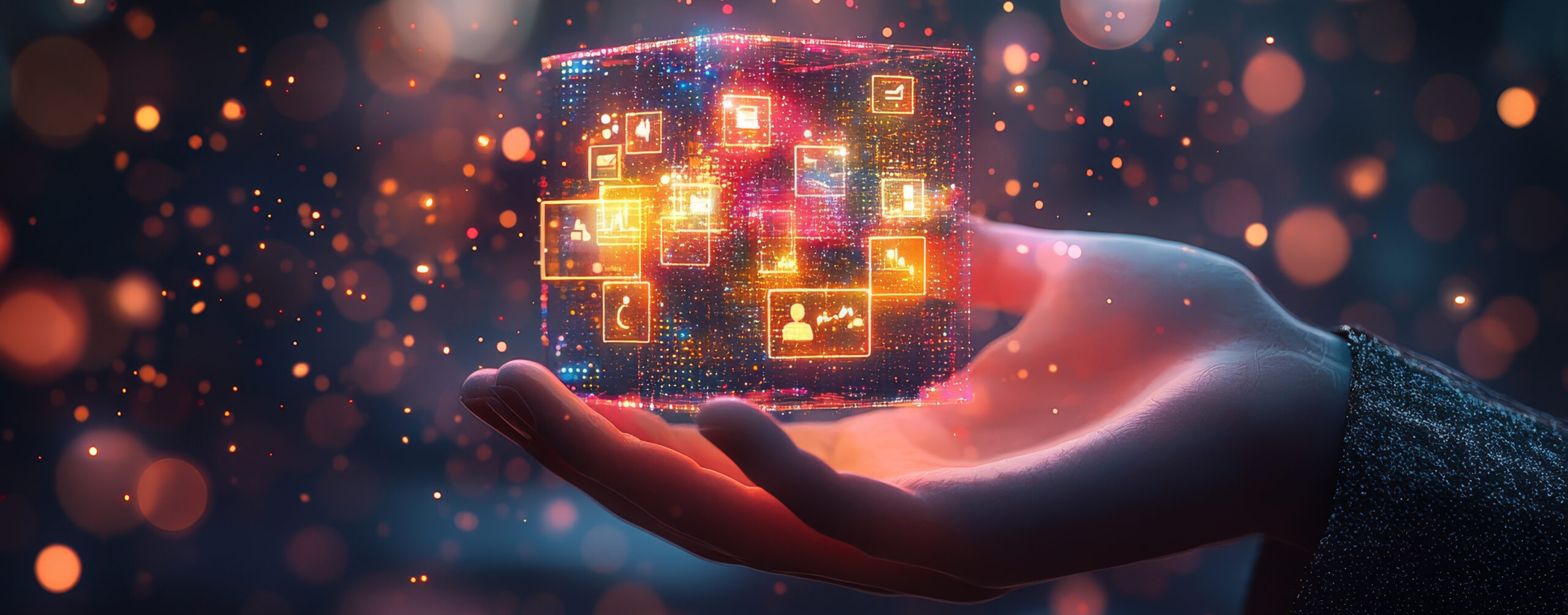
The Future of Decision Intelligence: What’s Next?
Emerging Trends in Decision Science & AI. The next evolution is Decision Intelligence, which integrates BI, BA, AI, robust technological methods, and human expertise to create a holistic, data-driven decision-making ecosystem. As AI and decision science continue to evolve, businesses that embrace these emerging trends will be better equipped to navigate complexity, reduce uncertainty, and drive smarter decisions in an increasingly data-driven world. Understanding both data/technology and human behavior will be key to unlocking better business outcomes. BI and BA are powerful tools, but their effectiveness depends on how well they align with human decision-making processes. By incorporating decision science and focusing on user experience, we can transform data into actionable insights that drive real-world results.Augmenting human intelligence with AI-enabled BI/BA visualization systems will empower businesses and drive confidence in your analytics-driven business decisions.
In the evolving landscape of analytics, Decision Intelligence represents the next step, one that blends technology, data science, and human psychology to empower us beyond traditional decision-making, fostering a more holistic and dynamic approach.
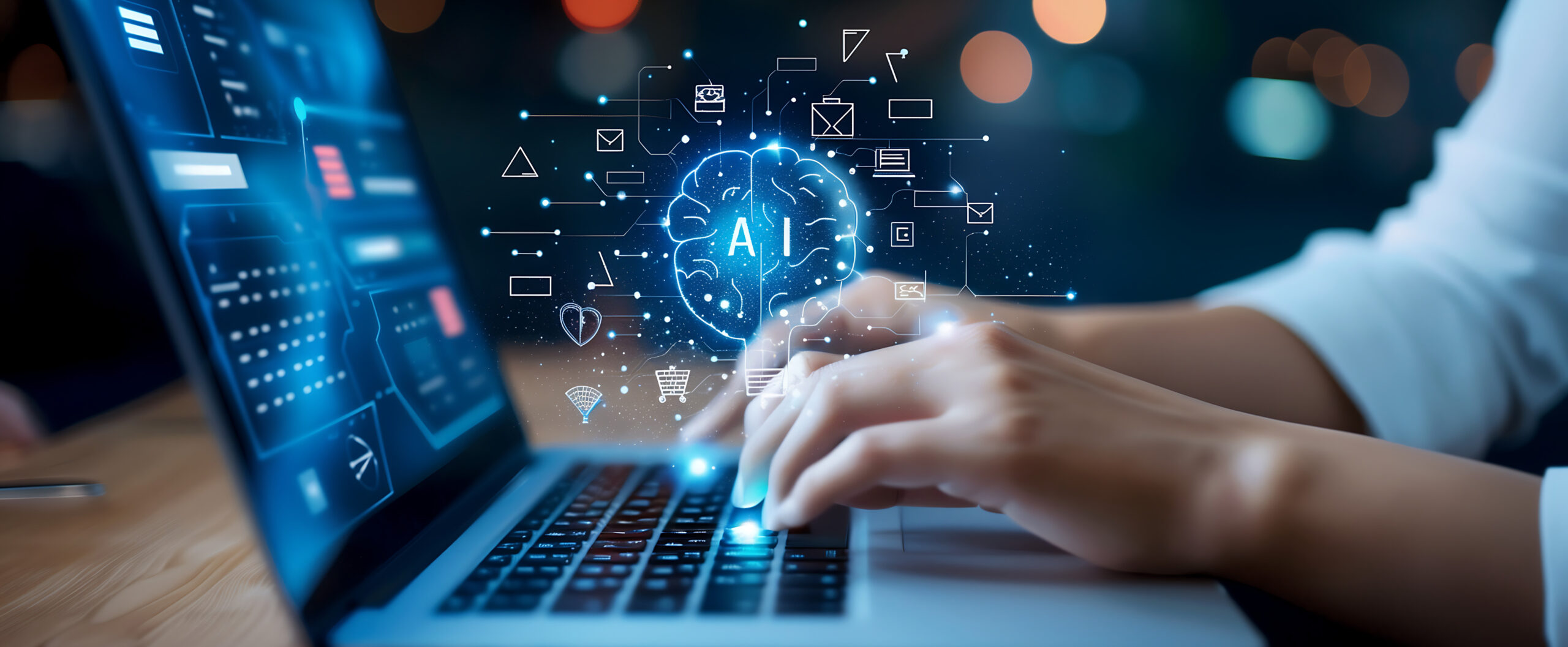
About the Author
Audrey Way. Audrey is a creative and thoughtful data visualization specialist adept at developing and optimizing dashboards in Tableau and Power BI to provide actionable insights, enhance decision making, and drive process improvements. She currently leads development and enhancement of dashboards on our Odin-FM project, ensuring high-quality data visualization that supports Air Force financial analysts and stakeholders in making informed financial budget planning decisions.
Audrey has experience leveraging AI integration within dashboards to implement predictive models that correct erroneous data, leading to improved forecasting and accuracy. She is highly collaborative and supportive of her team and is constantly looking for ways to grow and enhance her knowledge and skills.
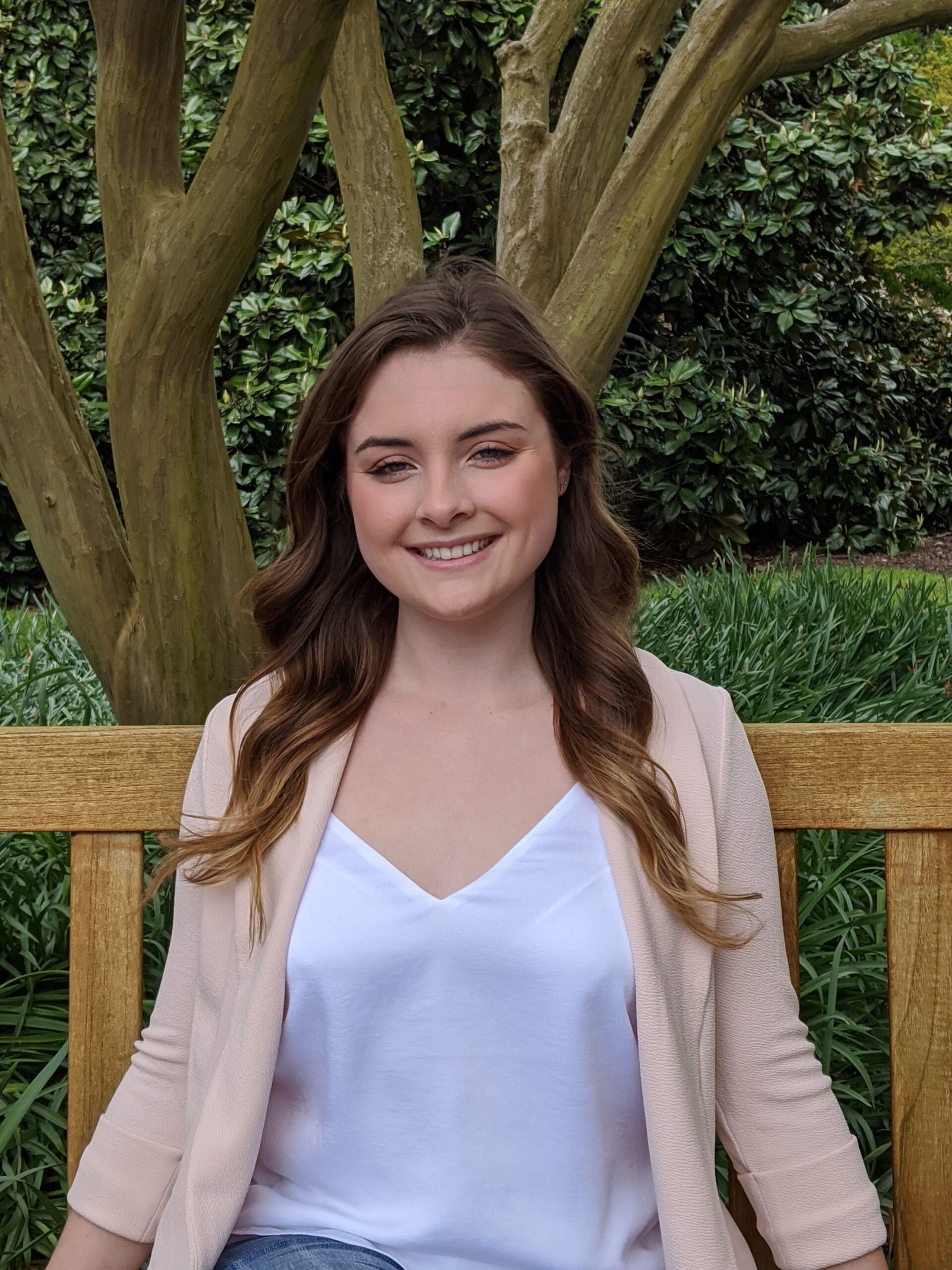
Special thanks to the contributors and technical reviewers of this article:
- Brandon Hurt, Senior Data Visualization Specialist
- Kristen Workman, Senior Software Developer
- Janette Steets, PhD, Director of Data Science
- Cathy Claude, Director of Marketing
If you liked this article, you might also like:
- Maximizing the full potential of AI with performance and scalability Read article
- Revolutionizing the AI landscape with retrieval-augmented generation (RAG), knowledge graphs, and quantized large language models (LLM) Read article
- Navigating the maze of AI cyber risks Read article
Sources referenced in this article:
- 73% of Business Leaders Believe Data Reduces Uncertainty and Drives Better Decisions — So Why Aren’t They Using It? – Salesforce
- What Is Business Intelligence (BI)? | IBM
- What is business intelligence? Turning data into business insights | CIO
- Pratt, L., Bisson, C., & Warin, T. (2023a). Bringing advanced technology to strategic decision-making: The Decision Intelligence/Data Science (DI/DS) integration framework. Futures, 152, 103217. https://doi.org/10.1016/j.futures.2023.103217
- The Psychology of Decision-Making: How We Make Choices | Psychreg
About Illumination Works
Illumination Works is a trusted technology partner in user-centric digital transformation, delivering impactful business results to clients through a wide range of services including big data information frameworks, data science, data visualization, and application/cloud development, all while focusing the approach on the end-user perspective. Established in 2006, the Illumination Works headquarters is located in Beavercreek, Ohio, with physical operations in Ohio, Utah, and the National Capital Region. In 2020, Illumination Works adopted a hybrid work model and currently has employees in 20+ states and is actively recruiting.
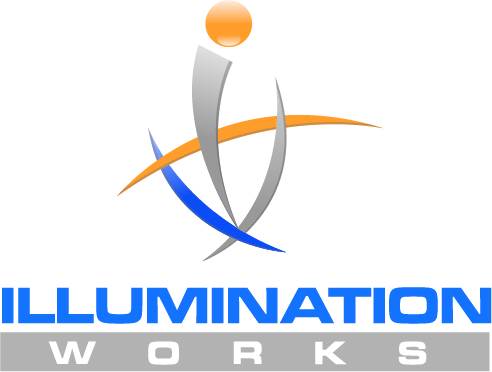