We sat down with our resident data solutioning thought leader, Gary Telles, VP Commercial Division, to talk about how remote autonomy based on extensive machine learning training and analytics at the edge can solve the communication challenge between a central data location and an edge device residing in a no/low bandwidth area.
What is the biggest communication challenge with edge analytics?
Low/No Bandwidth. The biggest communication challenge is that a remote device may only have the opportunity to communicate a few times a year. This low/no communication situation requires extensive training of an edge device prior to placing the device at the remote location, similar to a requirement to train a person that will be working at a remote location, both need properly trained to make decisions and function alone.
For a remote device, we can take artificial intelligence/machine learning (AI/ML) modules that are continuously improved in a lab through training and install that learning as a package on the device before deployment, or if while the device is remote there is an opportunity for sufficient bandwidth, transmit a new training module out to the device.
The installed training would consist of multiple AI/ML packages to collect situational awareness (externally and internally to the device), run analytics on the collection of information to determine what actions are needed, and produce additional analytics to prioritize outbound communication messages if the opportunity to communicate presents.
I like where Illumination Works is heading with pairing edge analytics with human-machine teaming–making a human plus device team at the edge. This pairing leads to improved human situational awareness, best recommendations for course of action, and reduced overall cognitive load. Pairing edge analytic devices with the human at the edge allows the human to focus on what really matters–doing their job efficiently and effectively.
With no/low bandwidth being one of today’s biggest challenges for edge analytics, extensive AI/ML training can solve this problem by enabling an edge device to act without communicating back to a central location for extended periods of time.
What are some examples that show the benefit of edge analytics?
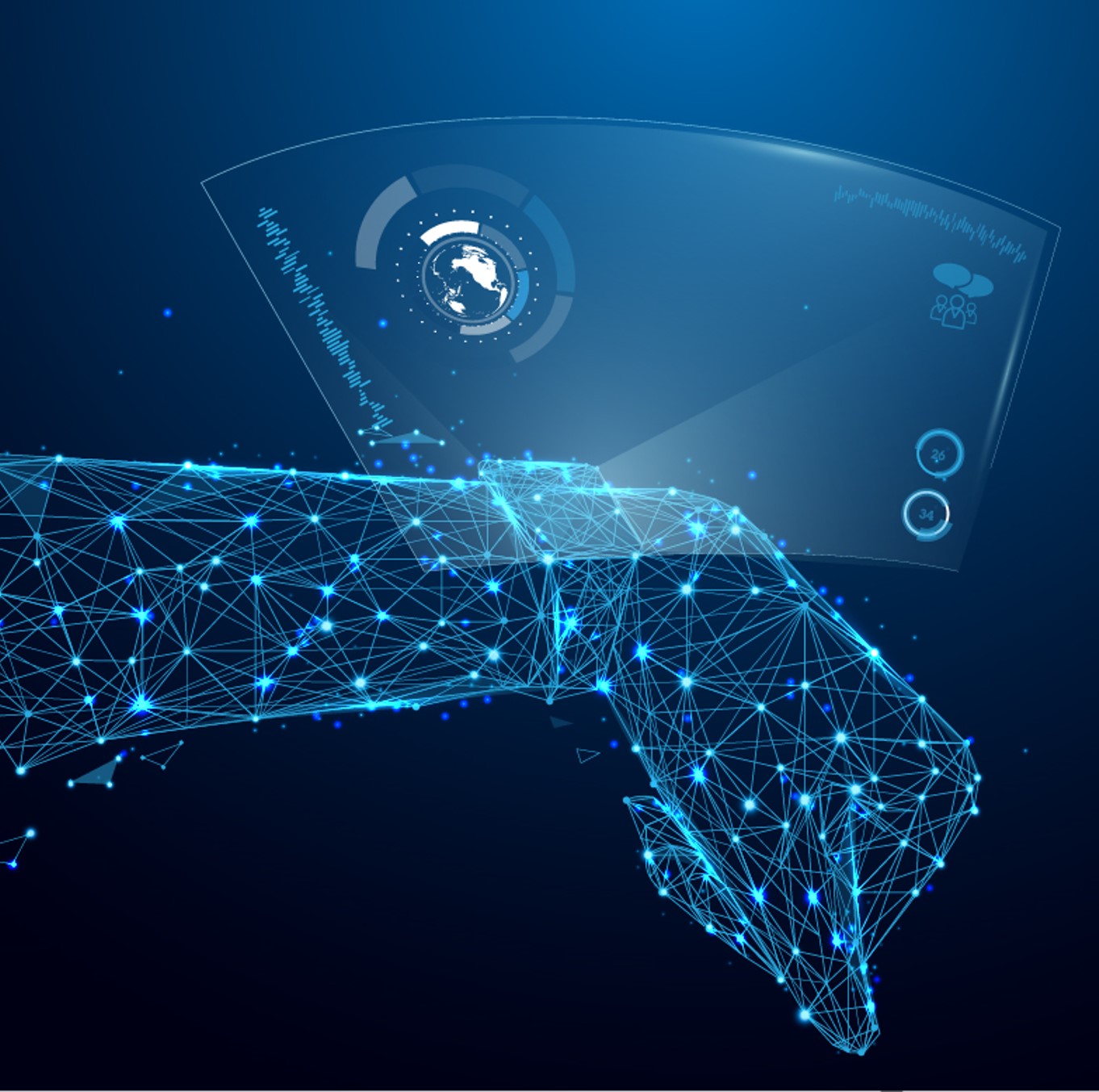
Fall Detection for Emergency Response. Extensive advanced AI/ML training condensed into small deployable modules are used in devices like smart watches to detect and discern the difference between jumping and landing versus an accidental fall. Where that accidental fall could require emergency assistance, the smart watch not only has detected the potentially harmful fall but has initiated a validation and alert sequence. If the watch wearer does not respond, then the harmful fall event was validated, and action will be taken by the device to alert emergency response with whatever communication options are available.
Condition-Based Maintenance. Another example is optimizing operations, maintenance, and inventory for condition-based maintenance for a globally dispersed, heavy equipment fleet with limited communications. Highly specialized operational maintenance teams can benefit from edge analytics when remote machinery can locally collect, detect, and predict potential failures “at the edge.”
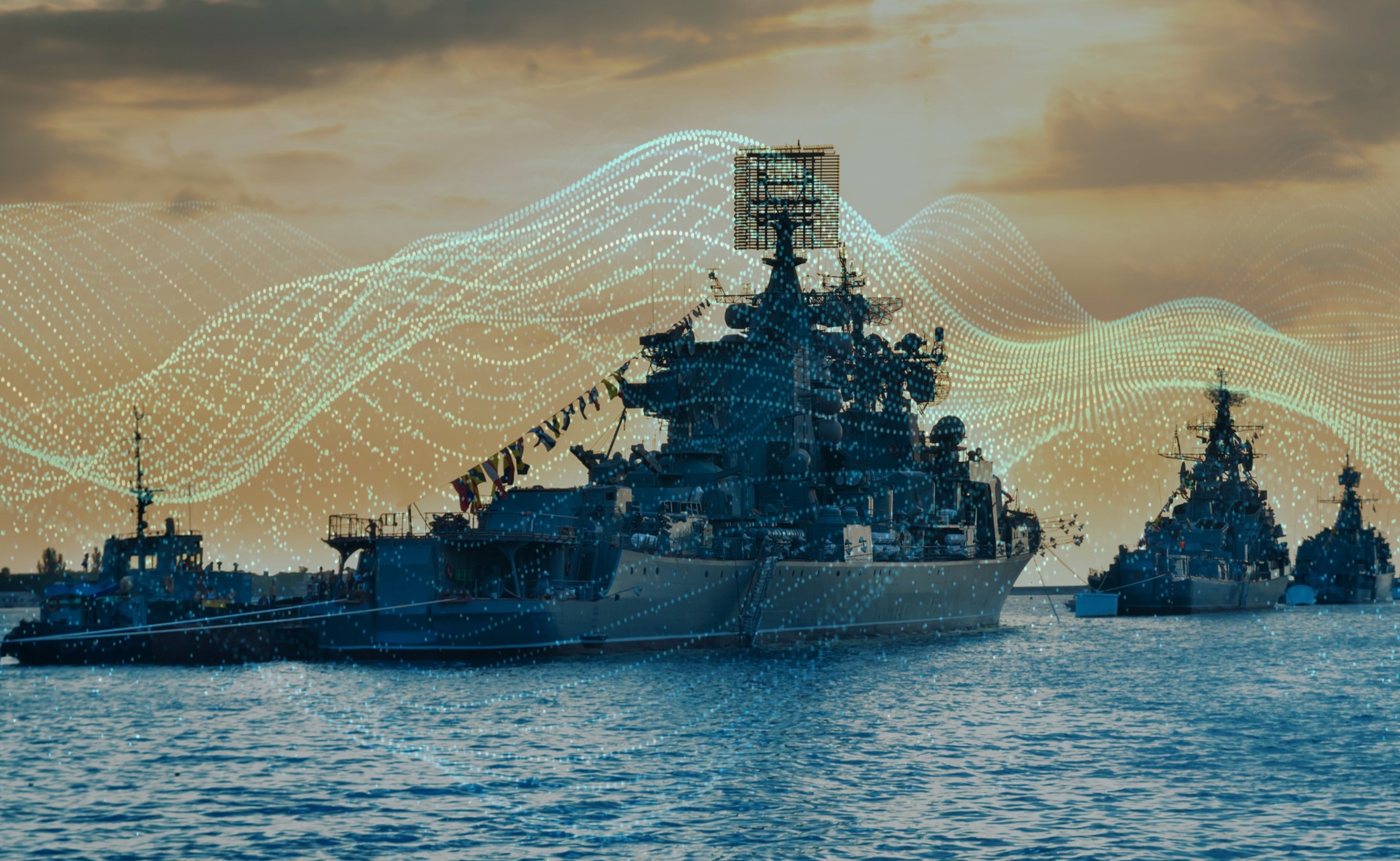
Time series data patterns of temperature, pressure, vibration, and other data are collected and analyzed together at the edge to forecast maintenance issues and associated time to failure. This list of forecasts and associated data evidence is saved for transmissions allowing the original data collection sensors to discard “normal” operating conditions if storage space becomes low.
Additionally, edge analytics eliminates the need for every sensor collection event to be communicated back to a central repository allowing machinery to operate in communication-challenged locations for extended periods. Once communication capacity is available, the central maintenance hub has a full picture of current and predicted future state of the remote machine.
What are the data capabilities of the central location versus an edge device location?
Central vs. Edge Location. The obvious difference is at a central location you have nearly continuous communication. This allows for additional resources including teams of data analysts and data scientists to offload the analytics from the edge to a central location. Another huge factor is the central location brings the big storage and compute power to the picture, whereas edge devices are limited to small storage and small compute power.
So, if we go back to an edge device that requires edge analytics, the challenge of saving massive data sets and acting on that collected data needs to be taken into consideration while training the edge device. This training will produce reliable analytics on collected data to take the best course of action as required or when opportunities present themselves.
Where are the data science activities and analyses performed in edge analytics?
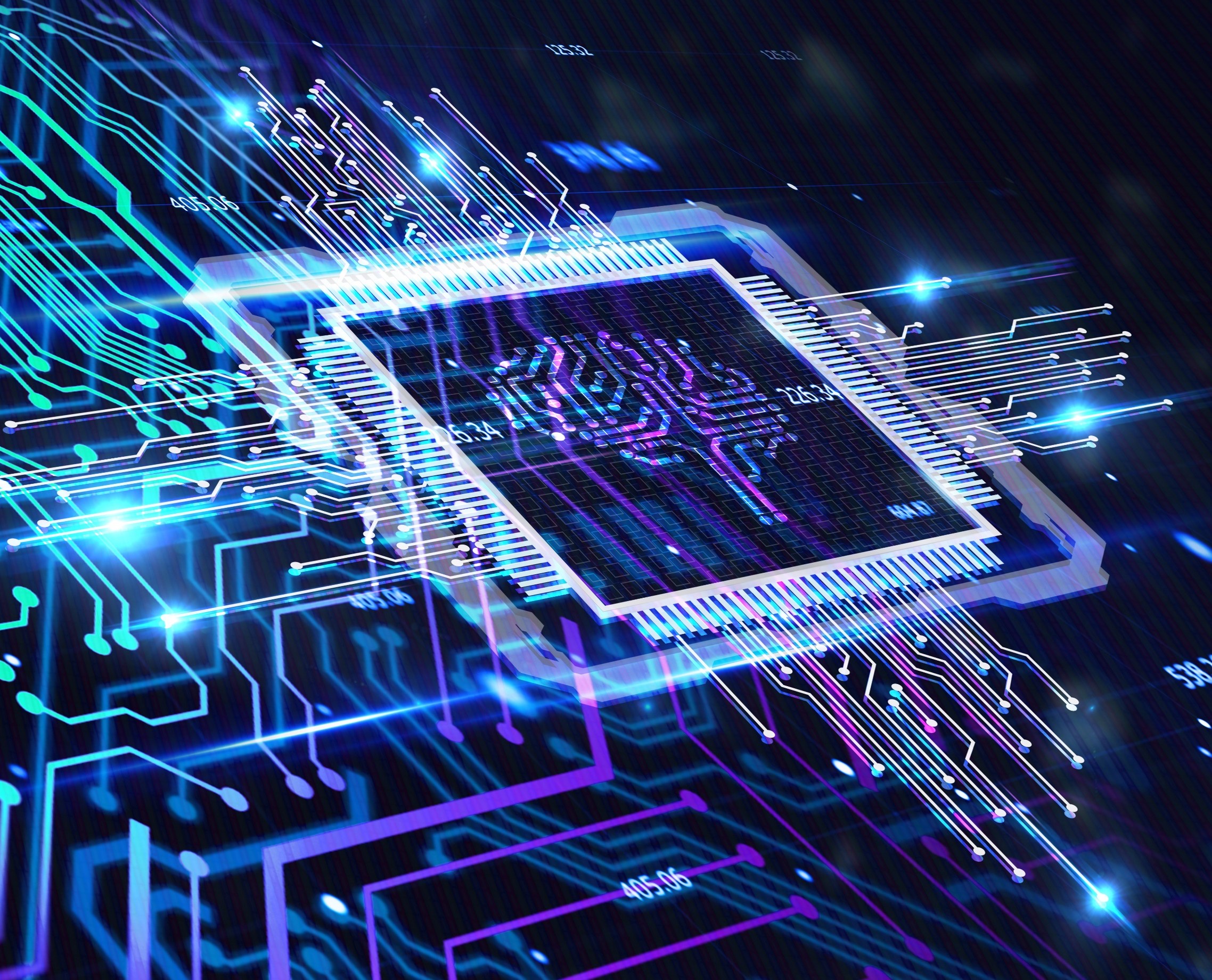
Training vs. Application of Model. Some activities are performed at the centralized location, and some are performed on the edge. Let’s decouple this into two distinct areas: The training of the model at the centralized location and the application of the model on the edge device in a no/low bandwidth location. To make analytics happen on an edge device with very small compute power, a massive amount of complex algorithm development and training is required by data scientists to build the ML model at the centralized location.
Once refined and tested, the model is minimized into small modules that are loaded onto the edge device, which is then physically deployed to the edge location.
The edge device collects all data in its surroundings and runs that data through the small machine learning models to analyze what observations meet the criteria set by the models. Then, the edge device, working with lower bandwidth availability, sends only a small amount of the full data set collected back to the central location.
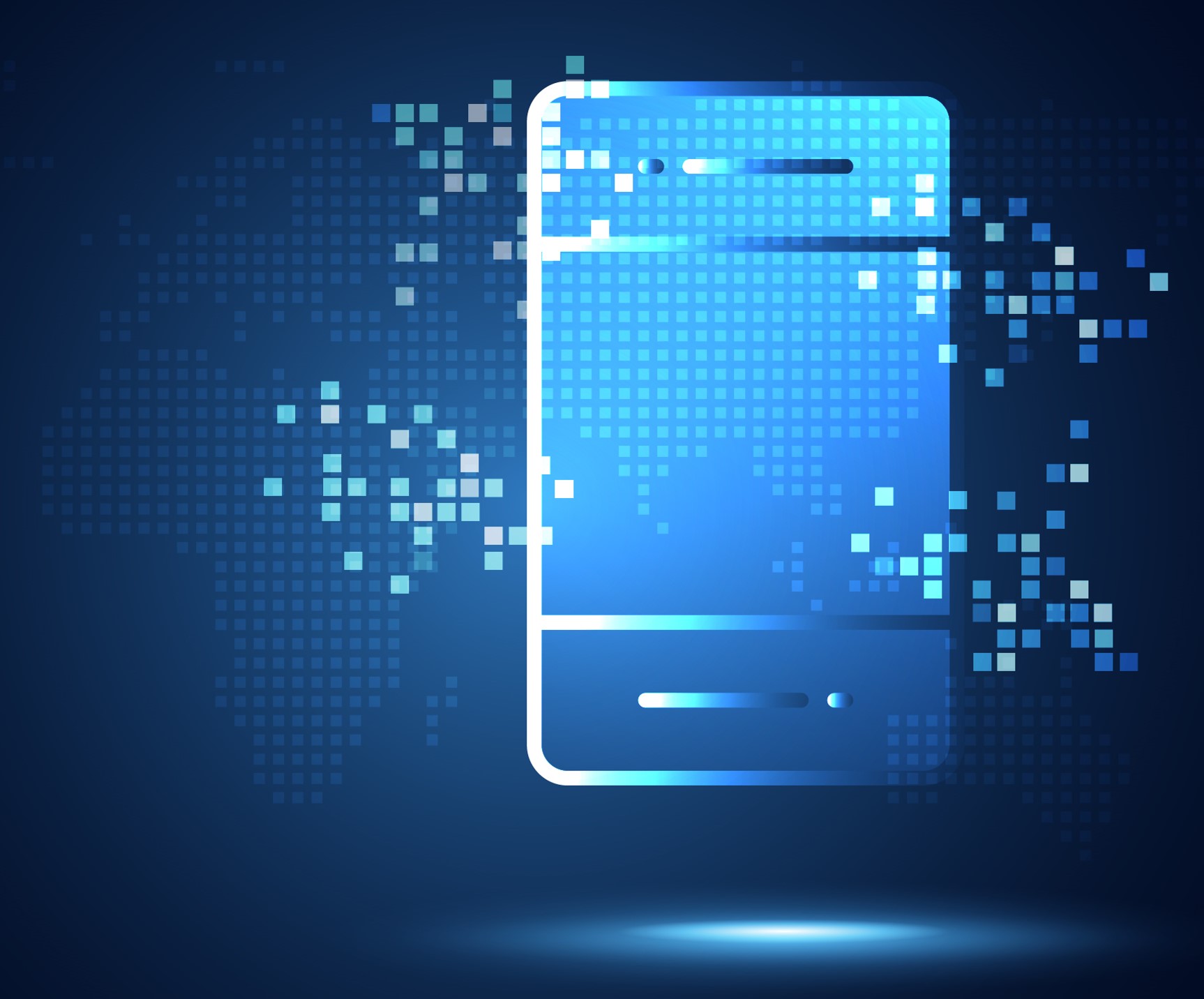
Can you delve a little deeper into the data modeling process?
![AdobeStock_236720373 [Converted] data modeling edge analytics blog](https://ilwllc.com/wp-content/uploads/2022/12/AdobeStock_236720373-Converted-data-modeling-edge-analytics-blog-2.jpg)
Bubbles & Cheese. Let’s use something abstract, like we want a device on the edge to identify things that look like bubbles and smell like cheese. To make this happen, the data scientists at the central location program the machine learning code to know what a bubble looks like and how cheese smells. This could include a large number of criteria that would need to be identified and trained. When the model is complete, the code is converted into a package of tiny training modules that are loaded onto a device so the device will know how to identify things that look like bubbles and smell like cheese in the edge environment.
Analytics at the Edge. Let’s say the edge device examined 400,000 things today, and found two things that look like bubbles and smell like cheese. Rather than transmitting all 400,000 things back to the centralized location, the device only sends the two things found based on the programming of the model. In a low bandwidth location, the device would not be able to send back all the 400,000 observations, but because the mini models are able to perform the analysis at the edge, the one-way transmission only needs to contain the two observations, solving the challenge of low bandwidth.
Action at the Edge. Not only is the model identifying certain criteria, but the model is also programed to perform an action when the criteria are met, which in this example, is to transmit the information back to the centralized location. Because the design is mostly a one-way communication, if incorrect information was being sent back, the model would need to be retrained at the central location and reloaded back onto the edge device. Similarly, if the device has low storage capability, the model could instruct the device to delete all 400,000 observations after transmission of the two observations, and then restart the observation period.
What personally excites you about edge analytics?
Edge Analtyics + Human-Machine Teaming. For me personally, I think where edge analytics is heading is exciting! I really like where Illumination Works is going in terms of innovation with human-machine teaming paired with edge analytics, making a human plus device team at the edge.
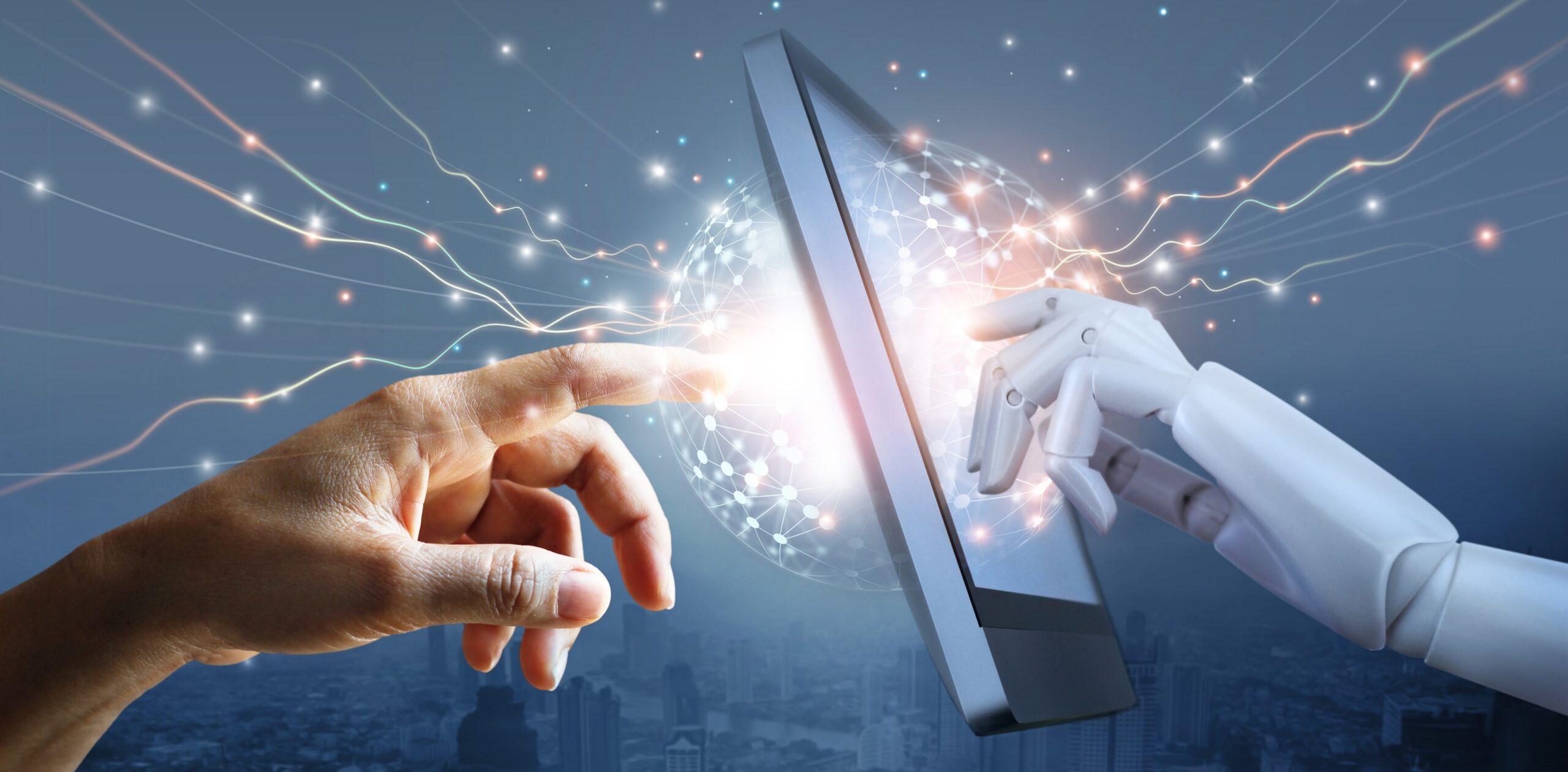
For example, adding several small edge devices supporting a human at the edge can improve a human’s situational awareness and present the best recommendations for course of action given what is happening or about to happen near that human. The end result is reducing the overall cognitive load and enabling the human to focus on what really matters, to work efficiently and effectively with all needed information at their disposal.
For questions regarding this blog, feel free to reach out to Gary Telles via email.
Check out our Edge Data Management & Analytics SBIR Phase I project.
Learn more about Kystone™ Edge Analytics and Data Resiliency solutions.
About Gary
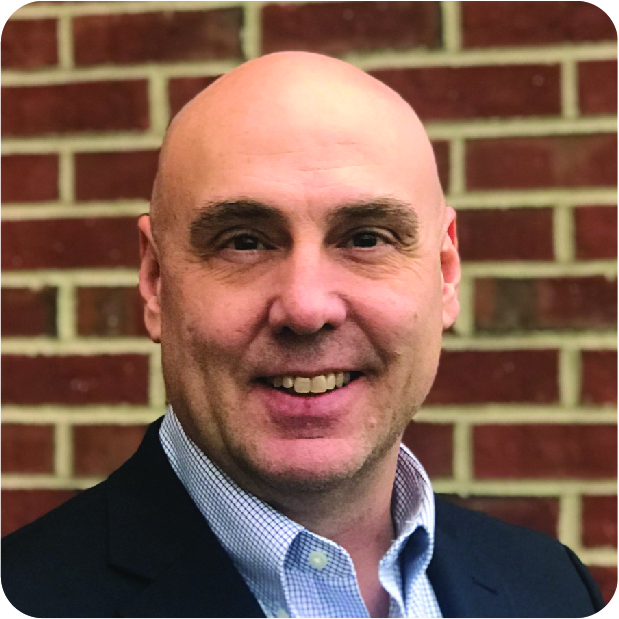
Gary Telles, VP Commercial Division, joined Illumination Works in January of 2010 and brings over 25 years of IT data experience leading data management, big data, data integration, data quality, and analytics projects. Gary continuously demonstrates thought leadership and innovation in data engineering/architecting state-of-the-art solutions for our clients. Gary is dedicated to bringing high quality, modern solutions to our customers and has a deep commitment to our people – always believing in, supporting, recognizing, and bringing together excellence in our talented team of data experts. Learn more about Gary on LinkedIn.
About Illumination Works
Illumination Works is a trusted technology partner in user-centric digital transformation, delivering impactful business results to clients through a wide range of services including big data information frameworks, data science, data visualization, and application/cloud development, all while focusing the approach on the end-user perspective. Established in 2006, ILW has offices in Beavercreek and Cincinnati, OH.
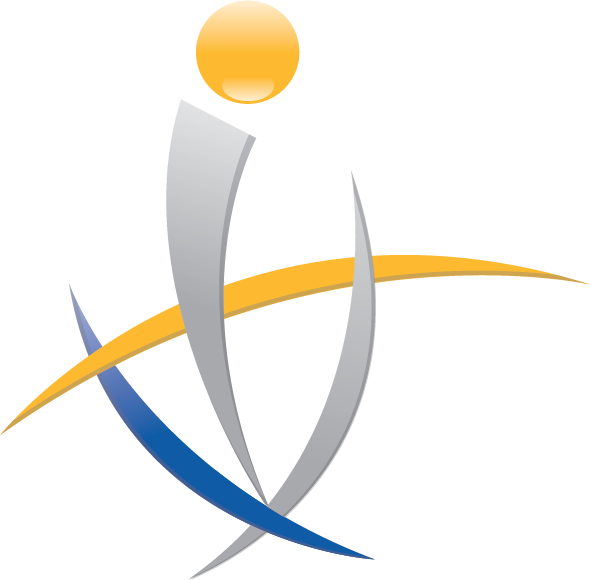