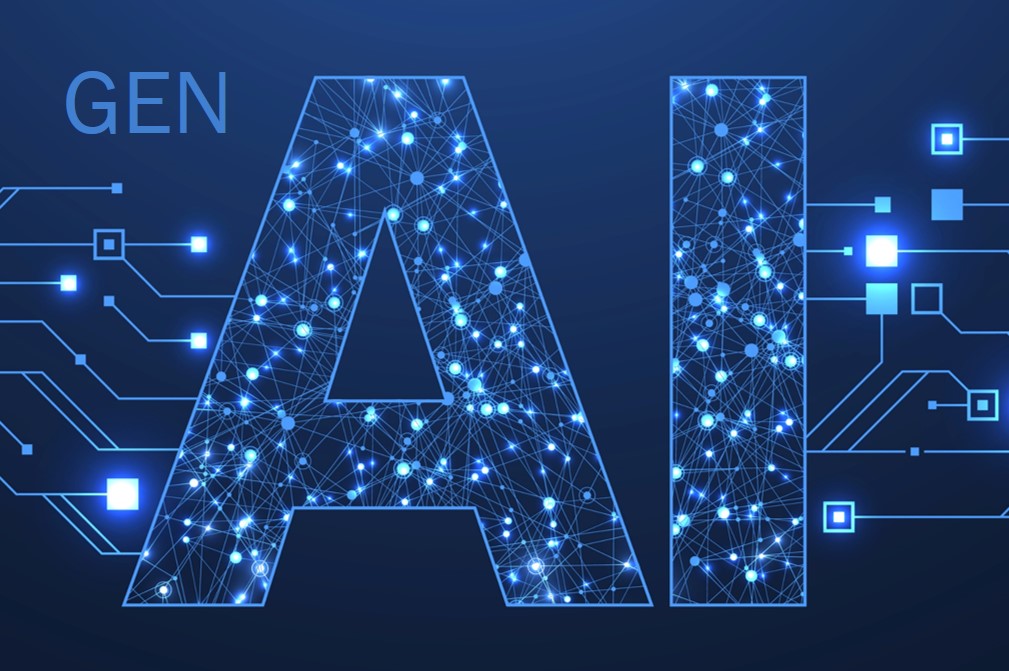
Generative AI for Predictive Logistics
Customer Challenge
The Air Force requires novel modeling process innovation to advance their logistics forecasting with the use of AI-generated maintenance data for “what if” alternative predictive scenario analyses.
Innovative Solution
Illumination Works developed a modular generative AI ensemble modeling methodology to create representative synthetic maintenance data for the future, based on past data. Our data science team created a pipeline of feature engineering, deep learning, machine learning, and generative AI methods to create generative maintenance data (GMD) to forge a new analytical path to predictive maintenance analytics. Our team validated the methodology on Air Force aircraft maintenance data, with the synthetic GMD data being statistically similar to the held-out validation data.
Benefits/Outcomes
- Allows for peripheral data science processes such as time series modeling to increase generated dataset accuracy and trends in the training data
- Provides unlimited scope with the ability to add any datatype to model/trend into the generated dataset
- Solution agnostic with relevant potential across other domains
Toolbox
- Data Engineering/Data Science
- Artificial Intelligence/Machine Learning
- Algorithm Development/Python
- Generative AI/Synthetic Data
- Forecasting
Business Value
- GMD provides an alternative approach to predictive maintenance for improved readiness
- GMD provides future data in the same form as historical data enabling users to plug in projections to existing code, reports, and dashboards to make decisions from it, as they would with historic data
- Saves money, time, and manpower
Domain Expertise
- Air Force weapon system maintenance data