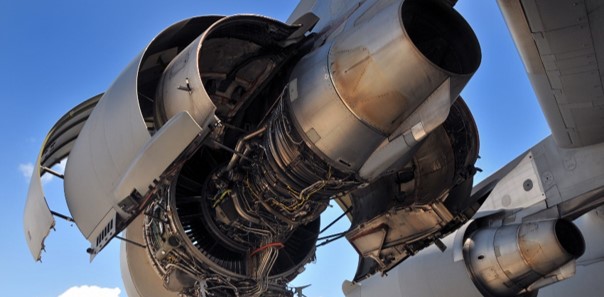
Automated Data Capture and Prediction
Customer Challenge
The Air Force required a method to modernize and ease airframe maintenance (Mx) data collection to improve usability, timeliness, and accuracy of data records.
Innovative Solution
As part of a Phase I SBIR, ILW data scientists extracted free text from Air Force maintenance transactions and mission debrief data to perform classification, training, and evaluation to statistically infer drivers and predict likelihood of occurrence of specific Mx actions based on correlations and ambiguities between free text and categorical variables to improve the accuracy of the data.
Phase II applied algorithm enhancements for improved entity extraction, classification, and prediction; created graphical and visual user interfaces for the maintainer; and produced outbound API to feed the Mx source system.
Benefits/Outcomes
- Simplified Mx action documentation required by Airmen
- Improved overall accuracy of enumerated codes
- Applied advanced text analytics to look at correlations between free text and categorical variables
- Improved timeliness and quality of Mx record
Domain Expertise
- Maintenance (Maintenance Narrative, Work Unit Code, HOW MAL Code, Action Taken Code, and other maintenance details)
Business Value
- Reduce maintainer time spent on documentation by 30-60 minutes per day
- Modernize the tools available to the workforce and reduce turnover
- Increase maintenance record accuracy by 10-30%
- Enable condition-based maintenance decisions
Toolbox
- Natural language processing, machine learning, modern workforce tools
- Predictive analytics, machine/adaptive learning, algorithm development, text analytics, entity extraction and classification, data modeling and federation, automated data pipeline